The Importance of Data Labeling in Machine Learning
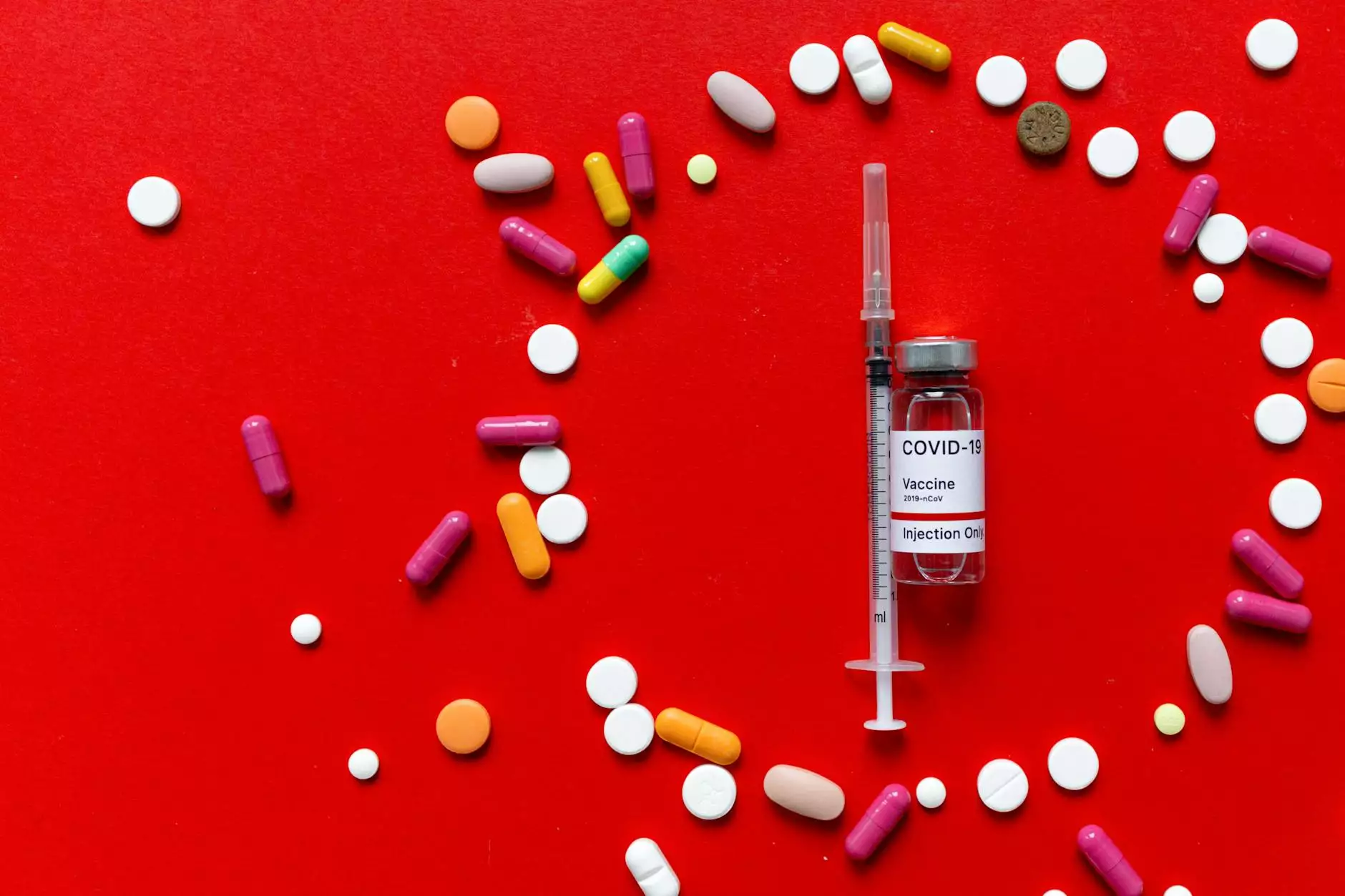
Data labeling is a foundational aspect of machine learning and artificial intelligence advancements. Businesses worldwide are increasingly recognizing its transformative potential. As we dive into this topic, we aim to provide a thorough understanding of data labeling and why it is integral to the success of machine learning initiatives.
Understanding Data Labeling
At its core, data labeling involves the process of tagging or annotating data to prepare it for training machine learning algorithms. This is a vital step because machine learning models learn from labeled data. When provided with accurate labels, these models can understand and predict outcomes based on input data.
What is Data Annotation?
Data annotation serves as the bridge that connects raw data to machine learning outcomes. It enables machines to take raw input—such as images, text, or audio—and convert it into a format that machine learning models can interpret. This process isn't merely about labeling; it’s about enriching data with context.
Types of Data Annotation
- Image Annotation: Marking objects within images, typically via bounding boxes, polygons, or landmark points.
- Text Annotation: Tagging parts of speech, entities, or sentiments within text data, essential for natural language processing tasks.
- Audio Annotation: Transcribing audio data, identifying speakers, or labeling specific sounds, crucial for speech recognition systems.
- Video Annotation: Recognizing actions or objects in video frames, making it suitable for video surveillance and action recognition applications.
The Role of Data Labeling in Machine Learning
The significance of data labeling in machine learning cannot be overstated. It directly influences the model's performance and accuracy. Here's how
- Guiding Model Learning: Machine learning algorithms require labeled data to learn effectively. The labeled data serves as the foundation upon which models identify patterns and make predictions.
- Reducing Errors: Proper labeling minimizes classification errors by ensuring data is correctly interpreted by the algorithm, leading to more accurate outcomes.
- Enhancing Model Generalization: High-quality labeled datasets help models generalize better to new, unseen data, which is essential for real-world applications.
- Facilitating Supervised Learning: In supervised learning—a commonly used machine learning paradigm—data labeling is essential. Without labels, training would be ineffective.
Challenges in Data Labeling
Despite its importance, data labeling presents several challenges:
- Time-Consuming: Manual data labeling can be incredibly labor-intensive, especially with large datasets.
- Human Error: Inconsistencies in labeling by human annotators can lead to poor quality datasets, which negatively impact model accuracy.
- Scalability Issues: As businesses grow, the need for larger labeled datasets increases, which can be difficult to manage using traditional methods.
- Cost Factors: Hiring skilled annotators or investing in data annotation tools can strain budgets.
Effective Strategies for Data Labeling
To overcome the challenges of data labeling, organizations should implement effective strategies:
- Use of Automated Tools: Leveraging automated data annotation tools significantly speeds up the labeling process. Tools can handle routine tasks, thereby reducing the burden on human annotators.
- Hybrid Labeling Approaches: Combining manual and automated processes can improve efficiency. For example, machine-generated labels can be reviewed and refined by human annotators.
- Creating Guidelines: Developing strict guidelines for annotators helps maintain consistency and accuracy across the labeled dataset.
- Quality Control Measures: Regularly auditing datasets can help identify mistakes and ensure high-quality annotations.
The Business Impact of Data Labeling
For businesses, investing in proper data labeling can lead to substantial benefits:
- Improved Accuracy: Models trained on high-quality labeled data yield more reliable predictions, leading to better decision-making.
- Faster Time to Market: Efficient data labeling processes enhance agility and speed up the deployment of machine learning models.
- Cost Efficiency: A streamlined data labeling process reduces costs in the long run, allowing for a better allocation of resources.
- Enhanced Product Development: With accurate machine learning models, businesses can improve their products, creating enhanced user experiences.
Choosing the Right Data Annotation Tool and Platform
When it comes to implementing a data labeling strategy, choosing the right data annotation tool or data annotation platform is crucial. Here are some key factors to consider:
- Flexibility and Scalability: Opt for platforms that can scale with your data needs and are adaptable to various project requirements.
- User-Friendly Interface: A simple, intuitive interface minimizes the learning curve for annotators and increases productivity.
- Integrations with Other Tools: Ensure that the annotation tool can seamlessly integrate with your existing workflows and tools.
- Security and Compliance: Consider the platform’s security measures, especially if you're dealing with sensitive data. It's essential to choose a compliant solution.
KeyLabs.ai: Your Partner for Data Annotation Success
At KeyLabs.ai, we specialize in providing exceptional data annotation tools and platforms that cater to the needs of businesses aiming to enhance their machine learning initiatives. Our robust solutions streamline the data labeling process, enabling efficient collaboration and high-quality outputs.
Our Services Include:
- Comprehensive Annotation Solutions: Offering services for image, text, audio, and video data, ensuring all your data types are covered.
- Customization: Tailored annotation guidelines and formats to meet your specific project requirements.
- Expert Teams: Our skilled annotators uphold meticulous standards to deliver high-quality labeled datasets.
The Future of Data Labeling in Machine Learning
As machine learning continues to evolve, the importance of effective data labeling will only grow. Emerging technologies such as artificial intelligence and deep learning are beginning to transform the data labeling landscape. With the integration of AI into data annotation tools, we are likely to see:
- Increased Automation: AI can take over repetitive labeling tasks, increasing efficiency and reducing costs.
- Improved Accuracy: AI-driven annotation tools can potentially lead to more accurate and consistent labeling.
- Real-Time Collaboration: Advanced collaborative platforms will facilitate seamless communication between teams working on data labeling tasks.
Conclusion
Data labeling is an essential component of successful machine learning projects, influencing the accuracy and robustness of AI models. As organizations work to harness the power of artificial intelligence, recognizing the value of quality data labeling becomes paramount.
By utilizing streamlined data annotation tools and platforms, businesses can overcome the challenges associated with data labeling while reaping the benefits of improved accuracy, efficiency, and ultimately, success in their machine learning endeavors. Partner with KeyLabs.ai for unparalleled data annotation solutions that position your business for future growth in the competitive landscape of AI and machine learning.
data labeling machine learning